Lessons from the Lovable Losers on Optimizing Your Test Engine
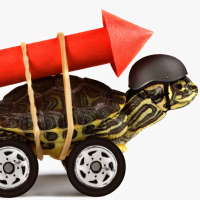
In many ways, the story of the Chicago Cubs and their improbable 2016 World Series championship can be traced back to the early 2000s. Competing for free-agent talent against big-money teams and armed only with scraps of a small-market budget, Oakland A's General Manager Billy Beane was in need of a competitive weapon. Help came in the form of a not-so-secret, decades-old concept, called sabermetrics.
The creation of statistician Bill James, sabermetrics takes an analytical view into baseball's rich statistical history to draw out nuggets of information tied to what matters most: relative performance against others and winning. By combining it with generous helpings of intestinal fortitude, Beane identified under-valued players that essentially flew below the radar of peers who relied on the conventional wisdom borne of traditional statistics.
Beane's success in applying these analytical insights to the team earned the A's playoff seeding in multiple seasons and elevated the team's story to pop culture status in the 2011 film Moneyball. The A's use of analytics also launched a front-office debate into the next decade between baseball's old guard executives and the newcomers armed with databases and spreadsheets. Fast-forward to 2016 when a shocked baseball nation watched the Lovable Losers win, which left many wondering, how? Led by General Manager Theo Epstein, another adherent to analytics, the Cubs’ championship signaled the end of the long-running debate on the use of analytics in Major League Baseball. Analytics had won.
The emergence of analytics in the business world has been just as swift. Predictive analytics has been churning behind the scenes managing our 401K investments and detecting fraudulent use of our credit cards. More recently, analytics and artificial intelligence have been making inroads in the business process automation of back-office functions such as accounting, legal, marketing, sales, and pricing.
As both the A's and the Cubs demonstrated, analytics provide insights by uncovering and making sense of the non-obvious, especially when partnered with a willingness to go against conventional wisdom. An engineering organization well-grounded in the benefits of test automation can naturally comprehend the business process automation qualities of speed, precision, and quality that analytics provides. For these engineering teams, analytics is quite possibly the next generation of test automation.
Which test organizations are well-suited for analytics? Which test processes could be targeted? And why is it important to pursue? The elemental resource for analytics is data. Engineering organizations that have matured their test automation capability are already generating reams of test results data. As Predictive Analytics author Eric Siegel states, "As data piles up, we have ourselves a genuine gold rush."
Some test organizations have started to apply analytics to processes such as test suite planning to optimize test regression runs, duplicate defect prediction algorithms to streamline defect triage efforts, and test configuration planning to reduce investment costs by identifying essential test configurations. Insight, speed, precision, and quality through an analytics-based competency are logical next steps for engineering organizations that already boast a test automation culture.
Geoff Meyer is presenting the session Leverage Big Data and Analytics for Testing at STARWEST 2017, October 1–6 in Anaheim, California, and at STARCANADA 2017, October 15–20 in Toronto, Ontario.